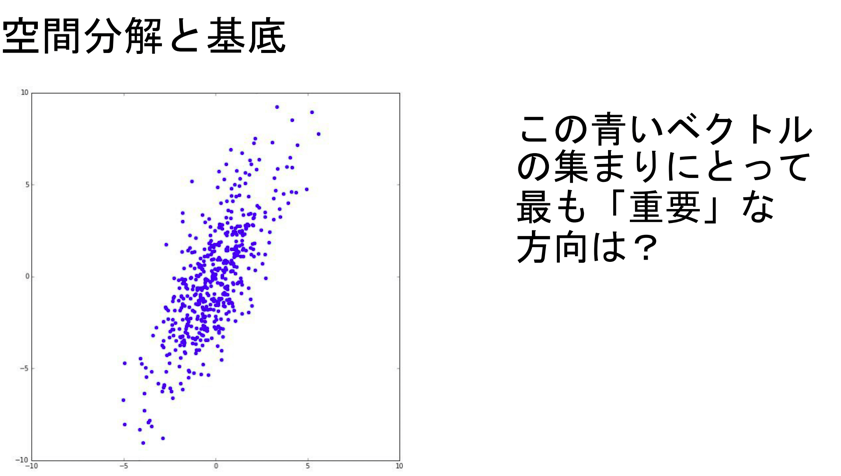
このデータは見ての通り2次元(平面)データです。これを1次元(直線)に置き換えたいです。
各点の配置は?
置き換える時に重要なのは距離感です。離れていた点通しが置き換えた先でご近所さんになると元のデータをうまく表現できてるとは言えませんよね。それが次の図です。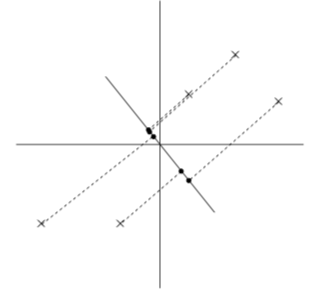
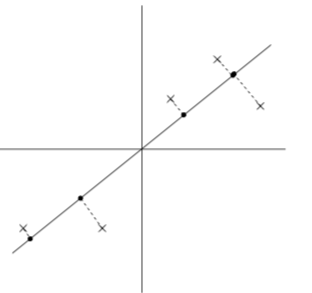
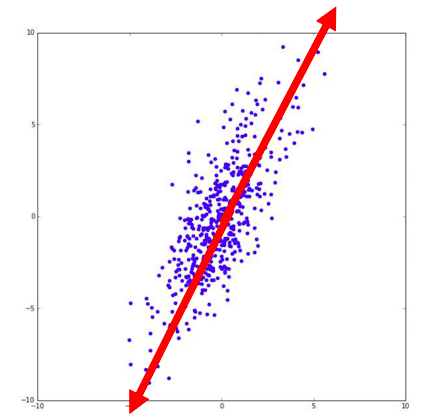

上の赤矢印(軸)
どうやっていい軸を選びましょうか。じーっと見てみましょう。広がっている方向に矢印が伸びていますね。つまりいい軸とはデータを矢印だけで表現したものと捉えることができそうです。では具体的にいい軸(ベクトル)をどう求めるかを考えましょう。 とりえあず求めるベクトルを


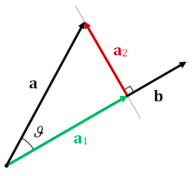




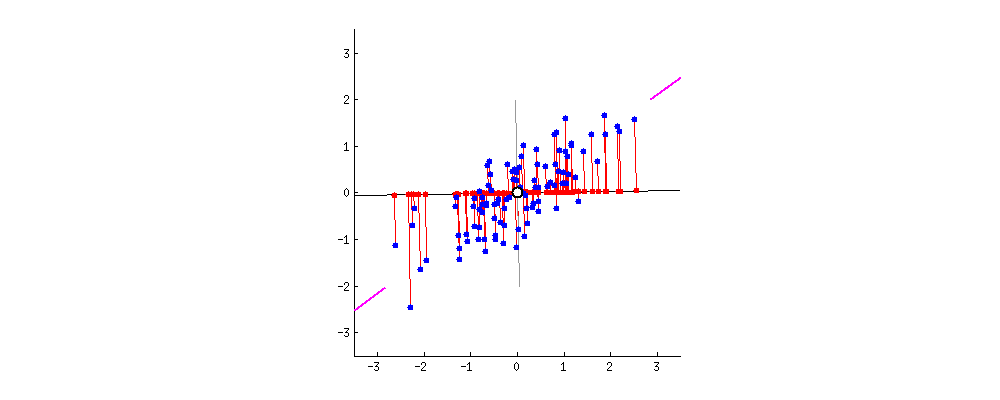








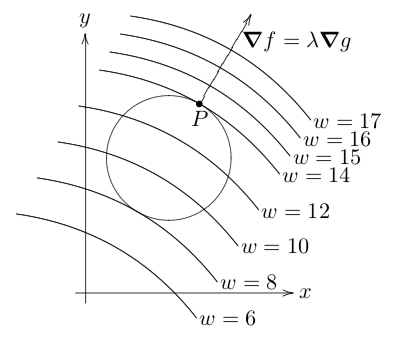
- https://en.wikipedia.org/wiki/Lagrange_multiplier
- http://www.yunabe.jp/docs/lagrange_multiplier.html
- https://ocw.mit.edu/courses/mathematics/18-02sc-multivariable-calculus-fall-2010/2.-partial-derivatives/part-c-lagrange-multipliers-and-constrained-differentials/session-40-proof-of-lagrange-multipliers/MIT18_02SC_notes_22.pdf
おっと、これわ。。。。
固有ベクトルと固有値そのもの
要点をいうと



- https://en.wikipedia.org/wiki/Eigenvalues_and_eigenvectors
- https://qiita.com/kenmatsu4/items/2a8573e3c878fc2da306
データの共分散行列の固有ベクトル
とわかりました。つまりデータを固有ベクトルという軸へ射影することで得た新たなデータ点はデータの次元を落としつつ最大限に元のデータを表現している新しいデータ
ということになります。これがPCAのやってることです。「PCAは射影である」
今回は2次元から1次元へのPCAでしたが一般にn次元へのPCAも同じです。固有ベクトルを軸としてとってきて射影により座標がデータのPCA後の座標です。2次元へのPCAなら2本の固有ベクトルにデータを射影して新たな座標を定義します。 最後に用語の説明をしておきます。- 第n主成分: 固有値が大きい方から数えてn番目のものに対応する固有ベクトルのことをいう。
- 寄与率: (第n番目固有値/固有値の総和)
- 累積寄与率: 寄与率の累積
寄与率?固有値?
はい、説明します。固有値は伸縮率といいました。つまり対応する固有ベクトル方向へのデータの散らばり具合です。よって固有値とはデータの広がり具合(分散)を説明します。なので「元データを説明している具合」を分散という観点から考えて寄与としています。 本日はここまで。でわ 参考;